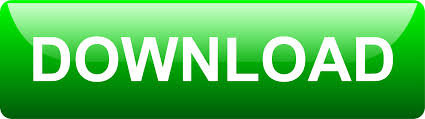
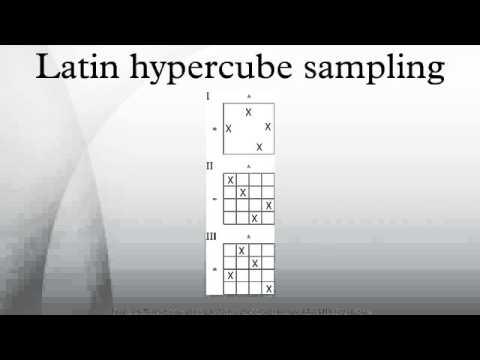
Other methods, such as Kriging and Bayesian emulation, have less dependence on the system’s smoothness and are more robust in that they do not require a specific set of simulations. Unfortunately, SC requires that all members of a specific deterministic set of simulations successfully execute. Some UQ methods, such as stochastic collocation (SC), exploit smoothness in the model’s output by using a set of samples to construct a fast surrogate for the computationally expensive simulator.
#Matlab latin hypercube sampling code simulator
When the simulator is sufficiently expensive, running one million simulations is unfeasible and faster methods are required. Because the error in a sample mean computed from a Monte Carlo sampling (MCS) design with N points scales as N -1/2, one million simulations will generally be needed for three significant figures of accuracy.

Randomly drawing samples from the distributions of uncertain inputs is one of the oldest, most robust, and universally applicable methods of uncertainty quantification (UQ). However, the correct input values for these models are often uncertain, and making accurate predictions of system behavior requires that this uncertainty be propagated to the models’ output. Models (i.e., simulators) are frequently used to make predictions about the performance of physical systems. KEYWORDS: uncertainty quantification, Monte Carlo, Latin hypercube sampling, space-filling, computational design, high-dimensional methods, regularity detection In anticipation of overcoming this obstacle, we outline a variant of the sorting algorithm with a low memory requirement for use in M ≥ 32 dimensions. However, memory requirements currently preclude even listing the 2 M / (2 M) orientation leaders for M ≥ 32 dimensions. Conceptually, the procedure works for arbitrarily large numbers of dimensions. In this paper, we use group theory to generate 2 M / (2 M) disjoint orientations, and present an algorithm to sort these into maximally spaced order. Even with this simplification, the ”patterns” for maximally spaced bins in M ≥ 16 dimensions are not so simple. Since the octants/bins containing the 2 M end points of an ”orientation” (a rotated set of orthogonal axes) are maximally spaced, the process of generating the list of octants simplifies to finding a list of maximally spaced orientations. Good Latin hypercube sample designs for non-power of two dimensions can be generated by discarding excess dimensions. For M = 2 r ≤ 8 dimensions and N = 2 s ≥ 2 M points, where r and s are non-negative integers, simple patterns can be used to create a list of maximally spaced bins.
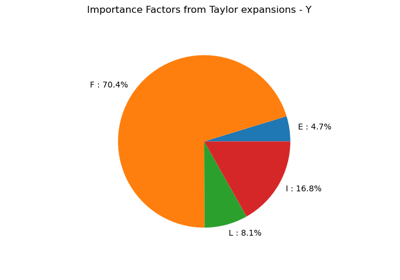
Specifically, Fourier analysis reveals regular patterns in the multi-dimensional spacing of points for the Sobol sequence but not for Binning optimal symmetric Latin hypercube sampling. Such designs are said to be ”binning optimal” and are shown to be irregular. A fast way to generate irregular space-filling Latin hypercube sample designs is to randomly distribute the sample points to a pre-selected set of well-spaced bins. However, such methods are time consuming and frequently produce designs that are highly regular, which can bias results. In the literature, space-filling Latin hypercube sample designs typically are generated by optimizing some criteria such as maximizing the minimum distance between points or minimizing discrepancy.
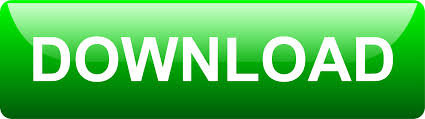